Executive Summary
Process Mining is an extremely useful tool in the practice of modern process automation. It not only helps to ensure processes and data align with company expectations but also can support organizations in maximizing process efficiencies and in helping companies focus on ways to improve customer service.
Despite the usefulness and applicability of process mining in most business processes, there are several major pitfalls that can severely handicap how well process mining works if not addressed properly, and the best time to do that is before implementation begins. In this blog we identify and describe five such challenges that new users of process mining need to make sure to avoid.
Keep in mind, process mining encompasses a tremendously growing and expanding field of automation techniques, so for customers considering use of process mining for the first time there is an ever-expanding set of features and techniques from which to choose. Of course, the critical consideration is which ones are the most beneficial for your company right now and in the immediate future. With the rate of change in the tool sets, it is unrealistic to factor in projections of the long term when it comes to process mining.
As noted, process mining tools are constantly under development and have ever expanding sets of major features and capabilities, including, most importantly, ever-increasing amounts of AI modelling and projections throughout the entire process mining framework. Because of this, it is critical that companies not be entirely dependent on the professional staff or partner consultants for the selected process mining tool. Company personnel need to be key contributors to the implementation of process mining on all a company’s processes. Companies need to take the significant step necessary to educate internal staff on the selected tool (and on process mining in general), to ensure that the tool succeeds and provides significant benefits to the company.
Here are some of the most common challenges companies face in their process mining initiatives. Since process mining tools are constantly being updated and new features and capabilities are being added, these particular challenges may lessen in importance over time and/or be replaced by new challenges due to advances in the tools.
Using Process Mining for a Process that Doesn’t Really Need It
While choosing the tool most appropriate for your company is a critical step, it is also important to analyze your own company’s processes thoroughly and take enough time to figure out where process mining can be best used and provide the most benefit. There are several signs in a process that can make it a very good candidate for process mining. These include overly complex data; no clear data structure apparent; unidentifiable problems, deviations and bottlenecks found in the process- just to name a few.
By picking a process that is not entirely in need of process mining, a company is essentially wasting its money for no important reason. The way to ensure that a process mining tool will be maximizing company benefits is to make sure to deploy it for a process that checks off the most boxes of being a good candidate.
Although using a process mining tool does not automatically correlate to maximizing a company’s profit, picking the most appropriate process to use the tool to start with will definitely increase a company’s chances at success and will greatly increase the internal momentum within the company to continue to expand the processes covered by process mining.
The Process Contains Incomplete Data
Having incomplete data in a process will be an obvious bump in the road towards achieving full process automation. Since process mining tools are specifically designed to find gaps in the data, using process mining for a process with incomplete data can make some sense in helping process engineers to better understand these gaps.
However, it will definitely be easier and more straightforward if these gaps can be filled before the process mining tool is implemented. Depending on which part of a process the data is missing, if there is missing data, the resulting process mining outputs may be significantly degraded as process mining tools produce a fully linked chain of events where each successive event impacts the rest of the actions. If data is missing in one part of a process, that will impede progress in gaining a full understanding of the process using a process mining tool.
The Process Has Too Much “Concept Drifting”
“Concept Drift” refers to the tendency of processes to change over time, in particular while they are under the ‘analysis microscope’ of the process mining tool. For example, process changes can occur during different seasons; this is an example of how processes can change periodically. The chart below demonstrates the various types of concept drifting that can occur in a process, whatever the underlying reason may be.
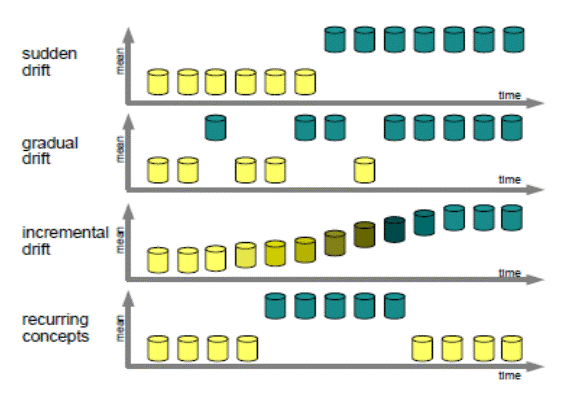
These drifts can impact the digital structure that process mining comes up with and will make the process mining analysis and results very unstable and difficult to interpret.
Splitting a process up into several smaller event logs is often an easy and effective way to discover possible concept drifting. Being aware of and managing concept drift in your process from the beginning before process mining is first implemented will help ensure that it remains controllable and understandable as process mining procedures are implemented, and prevent ‘drifts’ from having negative impacts on process mining analysis and results.
Data is Not in an Appropriate Format
Pre-processing and data preparation are vital steps to a successful process mining initiative and unfortunately this step is often overlooked. It is crucial to look at the import format of your data to ensure that it agrees with the process mining tool you have selected.
If there is a mismatch in format, the data will likely not be processed fully or even at all. In these cases, analyses from the process mining tools will be of poor quality, showing results with missing spots or even a completely blank event log.
Clearly this needs to be avoided, and you do that by preprocessing and adequately preparing the data for input to the process mining tool. Luckily, since process mining software is so diverse in its ability to work with most types of data sources, this challenge can be overcome if there is enough attention given to detail of the data inputs before the process mining tool is implemented. It is much better to deal with this issue ahead of time, than once process mining is already being implemented.
As you can see from the sample data below from QPR’s process mining software, the columns in this dataset are appropriately formatted and will be clearly readable to the process mining tool. Ensuring that data is neatly organized initially can save not only time but effort later-on so your company can focus on the major issues that process mining can help with, such as process deviations and bottlenecks.


Company Does Not Consider Overall Business Value Up Front
Before starting out with any process mining software, you should set a fixed objective of the overall business value the company wants to get out of implementing the process mining tool. Not highlighting and understanding what a company hopes to accomplish with its process mining tool can be a serious problem and will often lead to little to no enthusiasm within the company for continuing to pursue process mining long term for process automation.
Also, specifying which aspects of a process you wish to gain insights into can allow for a more streamlined experience during any process mining initiative. When starting out with process mining, some companies make the mistake of automating too generally and not focusing on any specific parts of their process. This is almost surely a recipe for failure of the initiative. it is critical to know and select the most relevant processes for initial process mining implementation.
It is also important to highlight the exact benefits that you anticipate will be improved from your process mining implementation, such as efficiency, cost, reliability, and or any other such values most important to a company. This can allow the company to move forward with more hypothesis-driven goals in order to deal with bottlenecks and process deviations one by one.
Conclusion
This blog is a quick overview of some of the major challenges that a company needs to identify and avoid in its implementation of a process mining tool. While companies often spend lots of analyst time and effort to evaluate and compare the different tools available in the marketplace, they often do not spend enough time recognizing and making sure to avoid the challenges listed above. We recommend you take a close look at these issues as well and make sure to avoid them ahead of time. We will be happy to work through these issues with you and ensure your process mining success in implementation. Give us a call!
ByImaan Ali, Ronald Mueller | Published on December 8th, 2020 | Last updated on September 6th, 2021 | Robotic Process Automation