With the accelerating improvement of NLP (Natural Language Processing) technology in recent years, the demand for speech recognition and analytics services has grown exponentially. Common use cases include video subtitle generators, voice-enabled virtual assistants, the smart speaker for home devices, and customer interaction analysis. Speech to text transcription is at the center of all these applications. Transcription accuracy is arguably the most important factor that determines the overall quality of the services.
This paper is the first of Macrosoft’s two-part series on Speech to Text quality assessment and analysis research on some of the leading tools available in the marketplace. Our focus is on contact center conversations where we took high-quality call recordings and fed them into the three leading speech to text platforms:
- CallMiner
- GCP (Google Cloud Platform)
- AWS (Amazon Web Services)
The evaluation metric we use is the BLEU (Bilingual Evaluation Understudy) score. The source audio we use is stereo mp3 format with 44100 Hz sampling frequency at 128 kbps bitrates, which is at the high end of contact center recording quality.
1 Background
1.1 Macrosoft’s Speech Analytics Services with CallMiner
Speech analytics services are one of Macrosoft’s six lines of business. We partner with CallMiner to take in multichannel customer interaction data including call recordings and chats in the contact center. Our certified data scientist team will harness the power of the CallMiner Eureka platform, combined with our expertise in machine learning and big data analysis. Macrosoft provides our clients with the most valuable and actionable insights to help your contact center improve as a business.
1.2 Amazon Transcribe from Amazon Web Services
Amazon Transcribe is the Speech to Text module provided by AWS, the market-leading cloud computing platform. Just like other services on AWS, Amazon Transcribe comes with an easy-to-use User interface to enable users to do transcription jobs individually or by large batch size. Custom models are supported where users can train the models using their data. We used the general model for this study.
1.3 Speech to Text from Google Cloud Platform
Unlike Amazon Transcribe, Google Cloud Speech to Text is API-based only without a user interface so developers are needed to use this service. Google provides an enhanced model specific for contact center conversation recordings. This gives significantly better results than the regular model, so we used the enhanced model for this study.
1.4 BLEU Score
BLEU (Bilingual Evaluation Understudy) is a metric for automatically evaluating machine-translated text. The BLEU score is a number between zero and one that measures the similarity of the machine-translated text to a set of high-quality reference translations. A value of 0 means that the machine-translated output has no overlap with the reference translation (low quality) while a value of 1 means there is perfect overlap with the reference translations (high quality)
2 Evaluation Method
To evaluate the transcript quality of the three platforms. We first manually create the transcription as ground truth by listening to the source audio. Then obtain three transcriptions after input the same audio to the platforms. We then compare the result and compute the BLEU score. We calculate BLEU at a sentence level and then used the average score across all sentences in the conversation as the final indicator.
The pictures below show the true transcript of a recorded sentence:


Below is the output from the three Speech to Text engines and their corresponding BLEU scores at the sentence level:


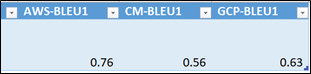
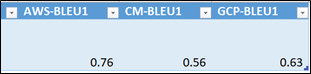
3 Result and Analysis


We repeat the process discussed in section 3 for each sentence and then compute the average score. The result is shown in the picture above. BLEU-1 is a unigram comparison, meaning we treat each word as a token and compare them one by one, BLEU-2 is a bigram comparison where two words form a token. The same logic applies to BLEU-3. BLEU-4 is a four-gram comparison plus a weighted sum of the previous BLEU score.
So, we generally look at BLEU-1 and BLEU-4 scores for the final result. If the order is the same for both. Then it’s safe to conclude we have a definitive result. Below is a reference chart for different ranges of Bleu scores (all numbers in the chart are percentages)


Looking at the result. All three platforms have very high quality transcriptions. The result is to be expected as the input data has very high bitrates. This shows that as long as we get good input data, the transcription quality will be very promising, which gives a strong base for the other applications that run on top of the transcript.
CallMiner and GCP outperform AWS transcribe as they use models trained specifically for contact center recordings rather than the generic model used by AWS. Each platform has its pros and cons and we will discuss them in detail in part two of this series.
4 Further Analysis, result by channels


In this part 1 of this study the source data is stereo recordings of the contact center calls. So we were able to take this study one step further to look at the transcription quality by channel. We can see the result is consistent across all platforms that the transcription quality of Agent speaking is much higher than the customer channel.
This result makes sense as in a call, the agents usually have professional background settings and an appropriate work environment, and the agent is trained to speak clearly. Meanwhile, the customer channel could be filled with many sources of noise such as a noisy background or a poor microphone and signal. In addition, the agent is trained to speak with appropriate tones and speed, which also helps the machine to pick up the transcription quality.
5 Summary
That concludes part 1 of our study. Part 2 will be published soon. As shown, for good conversational recordings, Call Miner performs consistently better than the two other speech to text analytics tools we evaluated from AWS and Google. To some extent this is not surprising since Call Miner technology is specifically honed and trained to analyze and interpret contact center conversations. As a dedicated Call Center implementation partner we are pleased to see that Call Miner technology maintains its leading position in contact center conversational transcriptions.
By Muhammad Saqib, Allen Shapiro, Ronald Mueller | August 19th, 2021 | CallMiner
Recent Blogs
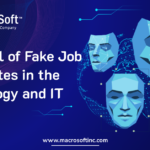
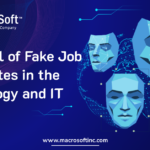
The Peril of Fake Job Candidates in the Technology and IT Industry
Read Blog

Humanizing Automation: Fostering Collaboration in the Digital Era
Read Blog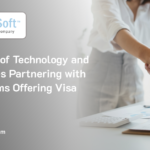
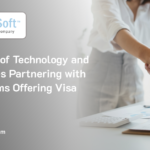
Advantages of Technology and IT Companies Partnering with Staffing Firms Offering Visa Sponsorship
Read Blog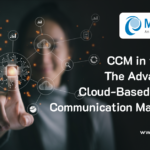
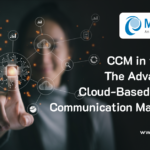