Macrosoft has completed a two-part research project comparing some of the leading speech to text tools available in the marketplace. This paper is the second of the series on speech to text quality assessment and analysis. Similar to part one, we fed conversational data from a contact center to the same three leading platform
- CallMiner
- GCP (Google Cloud Platform)
- AWS (Amazon Web Services)
In part one, the focus was the speech to text quality assessment for stereo high fidelity audio recordings. This time we choose to work with mono low fidelity audio. The source audio in this case is stereo mp3 format with 8000 Hz sampling frequency at 32 kbps bitrates, which is at the low end of contact center recording quality. The evaluation metric we use is the BLEU (Bilingual Evaluation Understudy) score, which is the same metric we used in the prior assessment.
Background
In the last paper, we briefly introduced CallMiner, GCP, and AWS speech to text services. Our study shows that all three platforms will give high quality transcripts when input audio is recorded at a high fidelity and stereo format. Further analysis shows that transcription of the agent’s channel is always better than the customer channel. Thus, we conclude the three key factors that determine the quality of speech to text transcription of a contact center conversation are:
- High quality stereo input recording data
- Speaking with a proper pace and tone
- Lessen background noise as possible
In this paper, we aim to further explore these conclusions by expanding our research to mono low quality input recording data. We will also review and discuss two important features that are highly demanded by customers these days: Redaction and Speaker Separation.
Results comparison and analysis

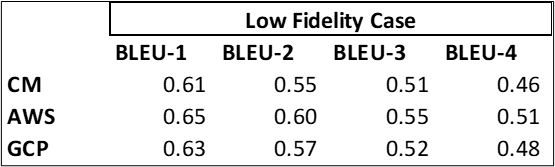
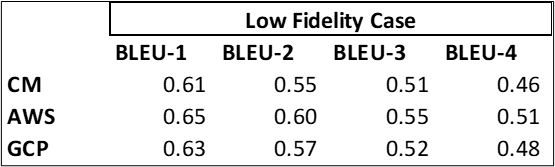
As shown above, all three platform’s transcription accuracy dropped significantly when input data is mono at low quality. This result is to be expected as the low fidelity data loses too much information about the original audio through sparse sampling or audio compression. Low fidelity data sometimes can even be hard for a human to recognize; thus the transcription engine will also have a hard time with it. Also, since the recordings are in mono, the transcription job becomes near impossible when there is overtalk in the conversations.
Feature evaluation
1 Speaker Separation
Speaker separation enables a speech to text engine to identify and separate two or more speakers in a single recording channel. This feature is very important for mono recordings of contact center conversations as speaker separation will identify the voices of the agent and customer. Having a transcript that’s separated by the speaker is much easier to read and understand than a mono transcript where everything is packed together. A speaker separated transcript is also necessary for reliable conversational analysis. All three platforms have speaker separation capability.
2 Redaction
Many contact centers are required to keep audio and text-based communication data. In these repositories, personal information is at risk due to a lack of redaction. All three platforms have redaction capability and CallMiner outperforms the other two services as CallMiner’s redaction can be applied on both the full transcript level as well as at the audio (word or phrase) level itself while GCP and AWS only do redaction at the transcript level. Furthermore, CallMiner provides two modes for redaction services:
- PCI Redaction redacts numbers when two or more numbers are found in the sequence. Any two numbers within one word of each other are redacted from both the audio and transcript.
- Targeted Redaction redacts numbers associated with specific keywords such as Social Security or Credit Card Numbers from both the audio and transcript. The table below shows the redaction details and the unique redaction code that’s searchable in the transcript to enable important speech analysis use cases. For example: Are my agents staying compliant when asking for customer’s credit card information?


Summary
Our study in this paper further proves that input data quality is the number one factor in a speech to text transcription accuracy. Unfortunately, most call center vendors record the conversation at a low rate with the mono format to save space and cost. We recommend that you switch your recordings to high fidelity stereo settings so it’s ready for speech to text transcription. Good transcription quality is the foundation of good conversational analysis, which will help give the organization a competitive edge in many fields such as contact center efficiency and customer satisfaction.
ByMuhammad Saqib, Allen Shapiro, Ronald Mueller | Published on September 16th, 2021 | Last updated on September 29th, 2022 | CallMiner